The 10th International Conference of the ERCIM WG on Computational and Methodological Statistics (CMStatistics 2017) will take place at the Senate House, University of London, UK, 16-18 December 2017. Tutorials will be given on Friday 15th of December 2017 and the CRoNoS Winter Course on Copula-based modelling with R will take place the 13-14 December 2017.
This conference is organized by the ERCIM Working Group on Computational and Methodological Statistics (CMStatistics), Birkbeck University of London and King’s College London. The new journal Econometrics and Statistics (EcoSta)and its supplement, the Annals of Computational and Financial Econometrics are the main sponsors of the conference. The journals Econometrics and Statistics and Computational Statistics & Data Analysis publishes selected papers in special peer-reviewed, or regular issues.
The Conference will take place jointly with the 11th International Conference on Computational and Financial Econometrics (CFE 2017). The conference has a high reputation of quality presentations. The last editions of the joint conference CFE-CMStatistics gathered over 1500 participants.
All topics within the Aims and Scope of the ERCIM Working Group CMStatisticswill be considered for oral and poster presentation.
Topics includes, but not limited to: robust methods, statistical algorithms and software, high-dimensional data analysis, statistics for imprecise data, extreme value modeling, quantile regression and semiparametric methods, model validation, functional data analysis, Bayesian methods, optimization heuristics in estimation and modelling, computational econometrics, quantitative finance, statistical signal extraction and filtering, small area estimation, latent variable and structural equation models, mixture models, matrix computations in statistics, time series modeling and computation, optimal design algorithms and computational statistics for clinical research.
Modeling time scale in high-frequency data
Abstract. The aim focuses on when and how time scale can be quantified based on high-frequency sample from a diffusion process. On the one hand, it is well-known that under the standard regularity conditions on the coefficients, the Gaussian quasi-likelihood efficiently works. On the other hand, however, one would get confused with the practical problem what value is to be assigned to the sampling stepsize, for there is no absolute correspondence between model-time and actual-time scales. In this respect, it should be noted that the sampling stepsize is a fine-tuning parameter affecting estimates. Although we could select it in a subjective manner to meet the theoretical requirements on the sampling frequency, it is obviously convenient to have a way to assign a specific value to the stepsize in a data-adaptive manner. We will propose a modified Gaussian quasi-likelihood function which is completely free from the stepsize fine-tuning, and also leads to the following desirable properties under an additional, seemingly non-standard identifiability condition. (1) The associated estimator is rate-efficient and asymptotically normally distributed. (2) The sampling stepsize can be estimated in some sense.
Speaker. Hiroki Masuda
Authors. Hiroki Masuda, Shoichi Eguchi
Hybrid estimators for ergodic diffusion processes based on thinned data
Abstract. Hybrid estimation is considered for both drift and diffusion coefficient parameters for discretely observed ergodic diffusion processes. In order to get the maximum likelihood type estimator, it is crucial to choose a suitable initial estimator for optimization of the quasi likelihood function. From a computational point of view, an initial Bayes type estimator of the diffusion coefficient parameter is given by using reduced data obtained from full data, and an initial Bayes type estimator of the drift parameter is obtained by using thinned data out of full data. The adaptive maximum likelihood type estimator with the initial Bayes type estimator, which is called hybrid estimator, is proposed. The asymptotic properties of the initial Bayes type estimators are proved. Moreover, it is shown that the hybrid estimators based on the initial Bayes type estimators have asymptotic normality and convergence of moments. In order to investigate asymptotic performance of the proposed estimators, we give a concrete example and simulation results.
Speaker. Masayuki Uchida
Authors. Masayuki Uchida
Lead-lag analysis of non-synchronously observed time series with R
Abstract. A lead-lag relationship is a time-lagged correlation structure of two time series wherein one is correlated to the other with a delay. It has also been well-recognized since long ago that the non-synchronicity of observation times of two time series causes a serious bias in estimating lead-lag relationships. To overcome this issue, a recent work has introduced a novel approach to compute the cross-covariances of the returns of two non-synchronously observed time series as well as proposed a simple statistic to measure their lead-lag relationship. The methodology is not only applicable to high-frequency financial data but also applicable to SNS data, so it could provide a useful tool for lead-lag analysis of time series to empirical researchers in any areas. R package yuima provides systematic functions to conveniently apply this methodology to real time series. The aim is to present them to empirical researchers as well as to show what we can really do in yuima. As an illustration, we will demonstrate its application to real data.
Speaker. Yuta Koike
Authors. Yuta Koike
The Yuima framework for simulation and inference of stochastic processes and its GUI
Abstract. The purpose is to present the Yuima package, a system of S4 classes and methods for the simulation and inference of stochastic processes including stochastic differential equation with or without jumps, fractional Brownian motion, Poisson and general point processes, CARMA and COGARCH processes. Yuima is a collaborative project and includes several simulation schemes as well statistical tools for quasi-maximum likelihood estimation, model selection, hypotheses testing, change point analysis. It also include methods of asymptotic expansion. Recently, the Yuima package has been coupled with a graphical user interface, namely the YuimaGUI, which simplifies the usage of the package and allows for a complete flow of analysis: from data ingestion, to model selection and or estimation, and estimation.
Speaker. Emanuele Guidotti
Authors. Emanuele Guidotti, Stefano Iacus, Lorenzo Mercuri
Curse of dimensionality of MCMC
Abstract. Some Markov-chain Monte Carlo methods for complex target distributions are studied and reviewed. We consider the effect of dimensionality along with the tail property of the target distribution. We also apply the results to the Bayesian inference for stochastic processes.
Speaker. Kengo Kamatani
Authors. Kengo Kamatani
New classes and methods in YUIMA
Abstract. Some advances in the implementation of advanced mathematical tools in the Yuima package are presented. First, we discuss a new class called yuima.law that refers to the mathematical description of a general Levy process used in the formal definition of a general Stochastic Differential Equation. The final aim is to have an object, defined by the user, that contains all possible information about the Levy process considered. This class creates a link between Yuima and other R packages that manage specific Levy process like for example ghyp or MixedTS available on CRAN. The second class, that we discuss, is the yuima.PPR that refers to the mathematical description of the Point Process Regression Model. This model can be seen as a generalization of a self-exciting point process, since it is possible to consider external covariates in the intensity process. We discuss the implemented methods for the definition, simulation and estimation of these processes.We also discuss the exact maximum likelihood estimation procedure for a Levy CARMA model. The new method is based on the existence of an analytical expression for the characteristic function of a general Levy CARMA model. We show that we are able to overcome the problems that arise in the estimation procedure based on Kalman Filter that requires the existence of the second moment for the underlying Levy process. Real and simulated examples are shown.
Speaker. Lorenzo Mercuri
Authors. Lorenzo Mercuri
Penalized methods for stochastic processes
Abstract. Quasi likelihood analysis (QLA) is a general framework for statistics for stochastic processes. The QLA established asymptotic (mixed) normality and convergence of moments of the quasi maximum likelihood estimator and the quasi Bayesian estimator for various dependency models such as diffusion processes, even under discrete sampling, and point processes. Recently applications of the QLA have been extending to regularization methods for sparse estimation. The penalized quasi likelihood function methods and the least squares approximation methods are discussed as well as their applications to volatility estimation for diffusion processes and a regression analysis for point processes.
Speaker. Nakahiro Yoshida
Authors. Nakahiro Yoshida
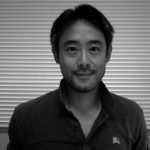
Hiroki Masuda
Full professor at Graduate School of Mathematics, Kyushu University. Research interests include inference for Lévy driven stochastic processes, stochastic approximation methods, ecology, forecasting energy-demand, and signal processing in life sciences.
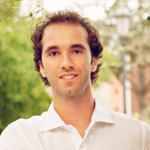
Emanuele Guidotti
Graduated in Physics (2015) and in Quantitative Finance (2017), both with honors at the University of Milan. Teaching assistant for course "Mathematics" at the Department of Social and Political Sciences. Now partner of AlgoFinance Sagl, software house start-up developing financial algorithms for the asset management industry, and Founder of the innovative start-up WhatsOut Srl, web application enhanced by AI. Developer of the yuimaGUI.
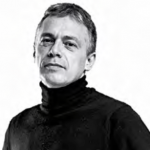
Stefano Iacus
Full professor of statistics the Department of Economics, Management and Quantitative Methods at the University of Milan. Member of the R Core Team (1999-2014) for the development of the R statistical environment and now member of the R Foundation. Research interests include inference for stochastic processes, simulation, computational statistics, causal inference, text mining, and sentiment analysis.
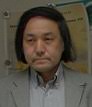
Nakahiro Yoshida
Full professor at the Graduate School of Mathematical Sciences, University of Tokyo. Working in theoretical statistics, probability theory, computational statistics, and financial data analysis. Awarded the Japan Statistical Society Award in 2009 and the Analysis Prize from the Mathematical Society of Japan in 2006.
You may also like
YSS2019: Computational and Statistical Methods for Stochastic Process
The first YUIMA Summer School on Computational and Statistical Methods for Stochastic Process This 4 days course aims at introducing researchers, PhD students and practitioners to several aspects of numerical and statistical analysis of time series…
Simulation and Inference for Stochastic Processes with YUIMA
Contains both theory and code with step-by-step examples and figures. Uses YUIMA package to implement the latest techniques available in the literature of inference for stochastic processes. Shows how to create the description of very abstract models in…
The YUIMA Project: A Computational Framework for Simulation and Inference of Stochastic Differential Equations
Abstract. The YUIMA Project is an open source and collaborative effort aimed at developing the R package yuima for simulation and inference of stochastic differential equations. In the yuima package stochastic differential equations can be of very…
COMPSTAT 2016, Oviedo
The 22nd International Conference on Computational Statistics took place at the Auditorium/Congress Palace Principe Felipe, Oviedo, Spain, 23-26 August 2016. The conference is sponsored by the European Regional Section of the IASC and is organized by the…